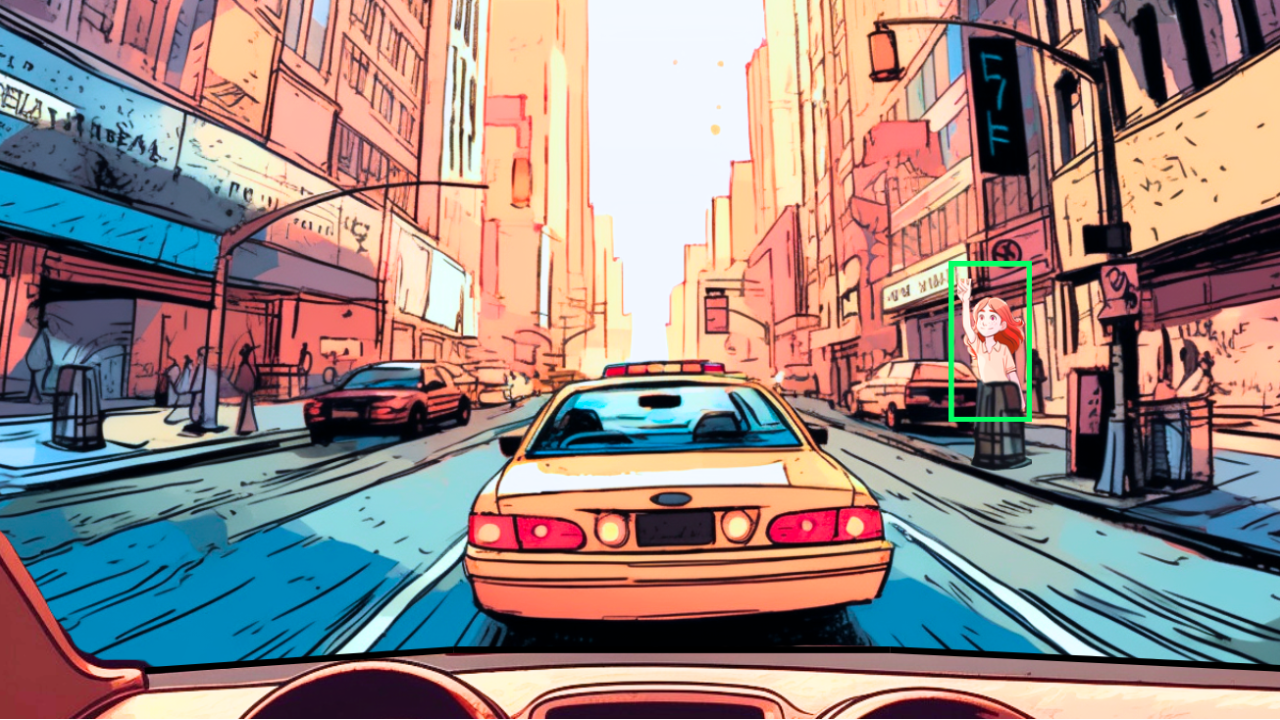
Driving Hazard Prediction by Multi-modal AI
- Post by: admin
- 2025-03-15
- Comments off
In recent years, with the advancement of autonomous driving technologies and advanced driver-assistance systems (ADAS), predicting hazards in the vicinity of vehicles has become a critical issue for safe driving. Conventional methods have relied on video analysis and simulations to detect anomalies over short time spans; however, this study adopts an approach similar to human reasoning—termed “visual abductive reasoning”—and proposes a novel framework that predicts future accident risks from a single still image.
Proposed Method
This study aims to estimate the likelihood of an accident occurring a few seconds later using only the limited information obtained from a dashcam still image and the vehicle’s speed. To achieve this, a multimodal AI technique that integrates both image and language information is utilized. Specifically, an open-source CLIP model serves as the foundation, and additional Transformer layers are introduced to fuse image and text features in a higher-dimensional space. This allows the method to capture the spatial and causal relationships among multiple objects, thereby realizing visual abductive reasoning.
Dataset and Evaluation Methods
Approximately 15,000 images, selected from existing dashcam datasets such as BDD100K and ECP, were curated to form the DHPR (Driving Hazard Prediction and Reasoning) dataset. Each image is annotated with the vehicle’s speed, a natural language description outlining the hazardous scenario, and up to three bounding boxes indicating the objects that contribute to the potential accident. The evaluation was carried out using tasks that involve retrieving appropriate hazard descriptions from images and generating these descriptions automatically, employing ranking metrics as well as evaluation measures such as BLEU, ROUGE, CIDEr, SPIDEr, and semantic assessments by GPT-4.
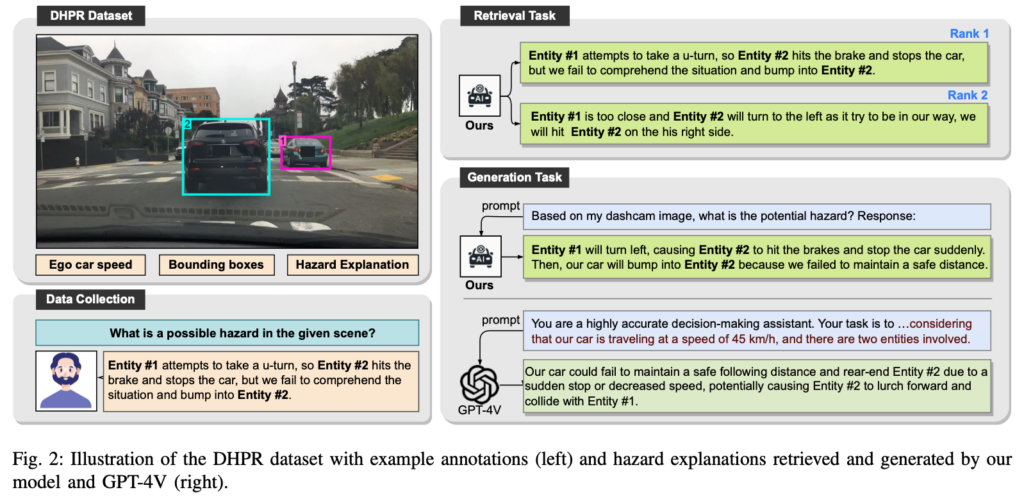
Experimental Results and Discussion
Experimental results show that the proposed model outperforms traditional BLIP-based models and other existing methods on hazard prediction tasks that integrate both image and text information. Additionally, ablation studies confirmed the effectiveness of each component—such as the robust visual encoder, the extra Transformer layers, and the entity shuffling method—in contributing to the overall improvement in prediction accuracy. Notably, despite relying solely on still images, the model is capable of predicting accident scenarios that closely resemble human intuitive judgments, highlighting its potential for practical applications.
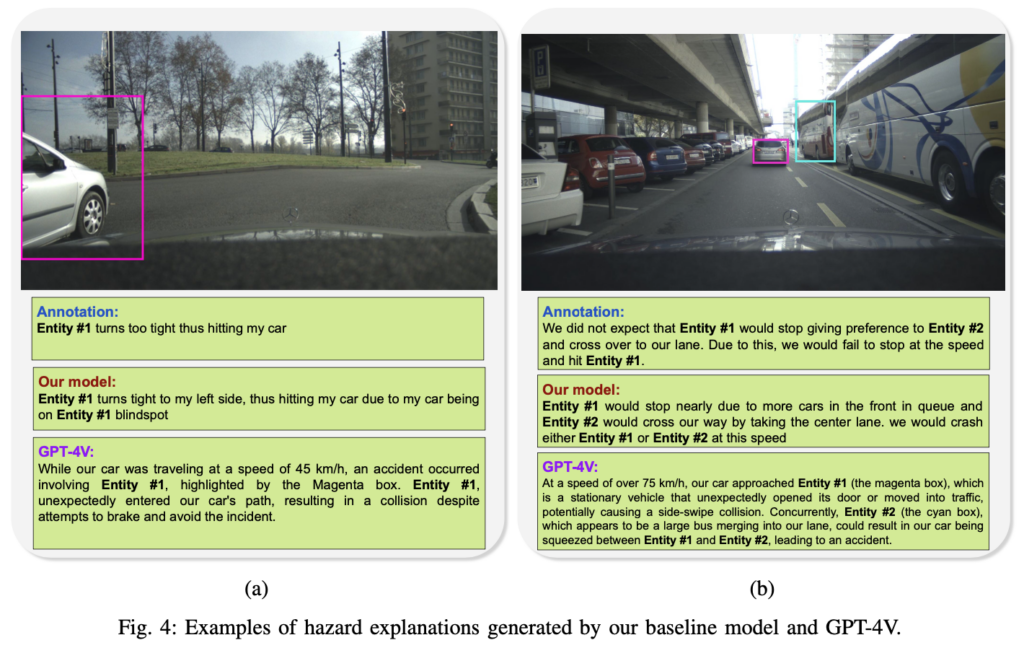
Conclusion and Future Prospects
This study presents a new approach based on visual abductive reasoning, which infers complex causal relationships from a single image—differing from traditional video analysis or simulation methods. By enabling the prediction of future accident risks with limited information, this method is expected to enhance the safety of autonomous driving and driver-assistance systems. However, integrating video and additional vehicular data for further performance improvement, as well as validating the system across diverse real-world scenarios, remain challenges for future research. Ultimately, the development of a comprehensive hazard prediction system utilizing more diverse input data is anticipated.
Publication
Charoenpitaks, Korawat, et al. “Exploring the Potential of Multi-Modal AI for Driving Hazard Prediction.” IEEE Transactions on Intelligent Vehicles (2024).
@article{charoenpitaks2024exploring, title={Exploring the Potential of Multi-Modal AI for Driving Hazard Prediction}, author={Charoenpitaks, Korawat and Nguyen, Van-Quang and Suganuma, Masanori and Takahashi, Masahiro and Niihara, Ryoma and Okatani, Takayuki}, journal={IEEE Transactions on Intelligent Vehicles}, year={2024}, publisher={IEEE} }