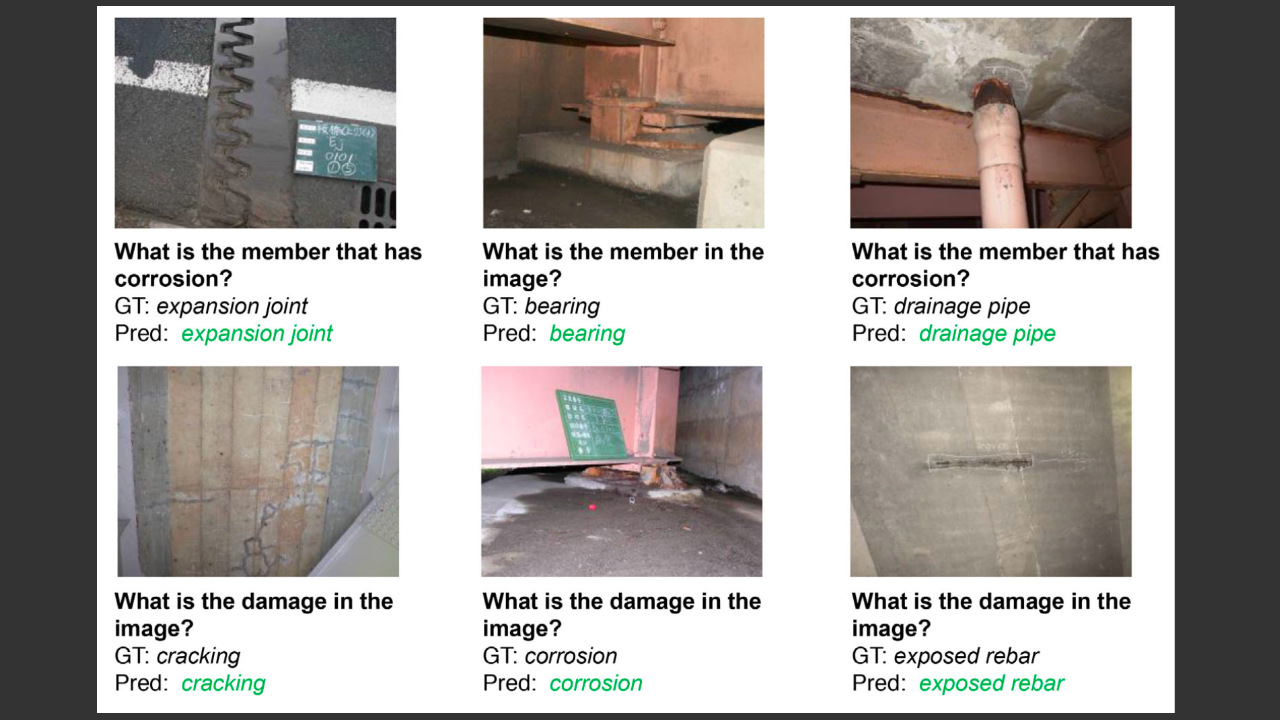
Bridge Inspection by Multi-modal AI
- Post by: admin
- 2025-03-16
- Comments off
This paper focuses on enhancing visual question answering (VQA) for bridge inspection using multimodal AI techniques that process both images and natural language. Traditionally, bridge inspections rely on expert visual assessments, which are time-consuming, costly, and sometimes inconsistent. To address these challenges, the authors propose a novel approach that leverages existing bridge inspection reports containing image–text pairs as external knowledge. By pre-training the VQA model with these pairs through Vision-Language Pre-training (VLP), the method achieves high accuracy even with limited task-specific data.
Related Work
Recent advances in multimodal models combining image recognition and natural language processing have produced remarkable results in general VQA and image captioning tasks. However, these methods have mostly been tested on everyday scenes or in fields like medicine, where ample data is available. In specialized domains such as bridge inspection, the collection of expert-annotated data is more challenging. Inspired by pre-training techniques applied in other specialized areas, this study employs VLP using image–text pairs to acquire domain-specific knowledge and improve model performance for bridge inspection.
Method
The proposed method adopts a two-stage learning strategy. In the first stage, a large collection of image–text pairs extracted from bridge inspection reports is used to pre-train the entire model. The image encoder is implemented using CNNs or Vision Transformers (ViT), while BERT is used as the text encoder. Pre-training tasks such as Masked Language Modeling (MLM), Image–Text Matching (ITM), and Image–Text Contrastive Learning (ITC) are combined to effectively capture the relationships between images and text. Additionally, the approach leverages models like MMBERT and ALBEF, and incorporates image encoders based on CLIP to extract bridge-specific features that are often overlooked by general pre-training methods.
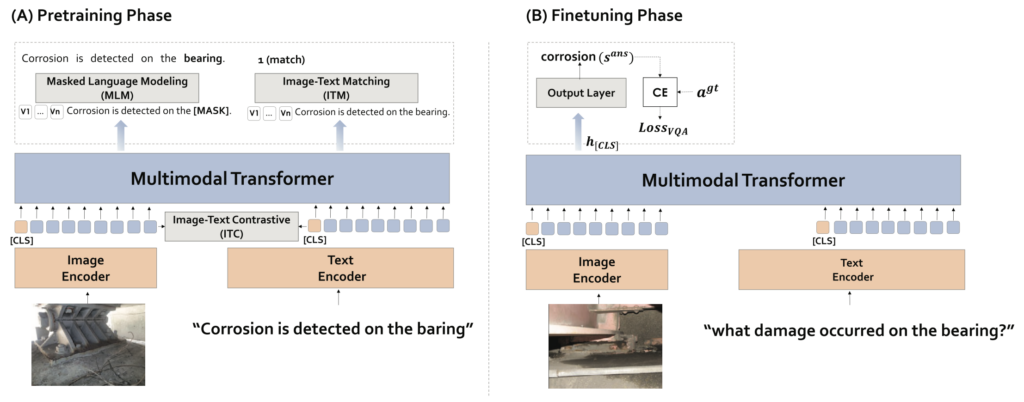
Experimental Results
Experiments were conducted using a large-scale dataset restructured from bridge inspection reports for image captioning, followed by fine-tuning on a dedicated VQA dataset. The results indicate that models incorporating the VLP stage significantly outperform baseline models in identifying bridge components and damage types. In particular, the introduction of Image–Text Contrastive (ITC) learning improved the accuracy of member and damage classifications by over 10%. The study also examines several failure cases, such as class imbalances and difficulties in recognizing close-up images, highlighting areas for future improvement.
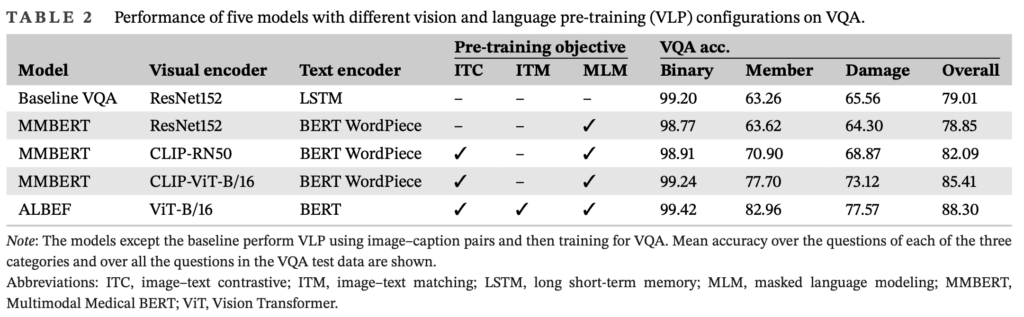
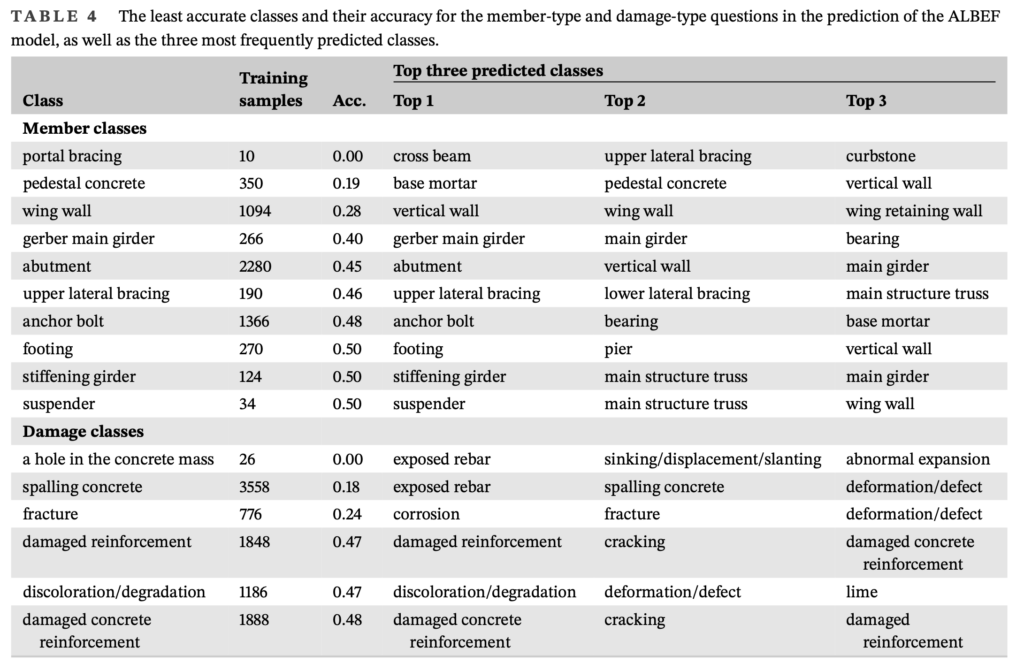
Conclusion
This study demonstrates that pre-training VQA models with external image–text pairs is an effective strategy for bridge inspection tasks. The proposed approach enables high recognition accuracy even with limited task-specific data, opening up possibilities for automated report generation and autonomous inspections using unmanned aerial vehicles (UAVs). Future work will focus on enhancing data quality and quantity, as well as conducting a detailed analysis of misclassification causes to develop more robust and practical systems, potentially extending the approach to other infrastructure domains.
Publication
Kunlamai, Thannarot, et al. “Improving visual question answering for bridge inspection by pre‐training with external data of image–text pairs.” Computer‐Aided Civil and Infrastructure Engineering 39.3 (2024): 345-361.
@article{kunlamai2024improving, title={Improving visual question answering for bridge inspection by pre-training with external data of image--text pairs}, author={Kunlamai, Thannarot and Yamane, Tatsuro and Suganuma, Masanori and Chun, Pang-Jo and Okatani, Takayaki}, journal={Computer-Aided Civil and Infrastructure Engineering}, volume={39}, number={3}, pages={345--361}, year={2024}, publisher={Wiley Online Library} }